In human visual system, capturing the scene gist is important for quick and exact scene classification and actually we are very good at getting the scene gist. This is a great challenge to vision researchers, given the complex structure of our visual world, and given the diversity of viewpoints that we can have on the world. Many researches and ideas have been proposed and enjoyed great success for categorizing objects, and this research focuses on two principle questions for scene gist model: how to build biologically relevant spatial layout of a scene, including both the choice of low-level features and the way to configure them spatially; and how to determine the resolution of the spatial layouts according to the given task.
As a result, a biologically inspired task oriented gist model (BT-Gist) that attempts to emulate two important attributes of biological gist: holistic scene centered spatial layout representation and task oriented resolution determination is presented.
The core of the BT-Gist, as shown in Fig. 1, concentrates on the biological plausibility and task oriented mechanism.
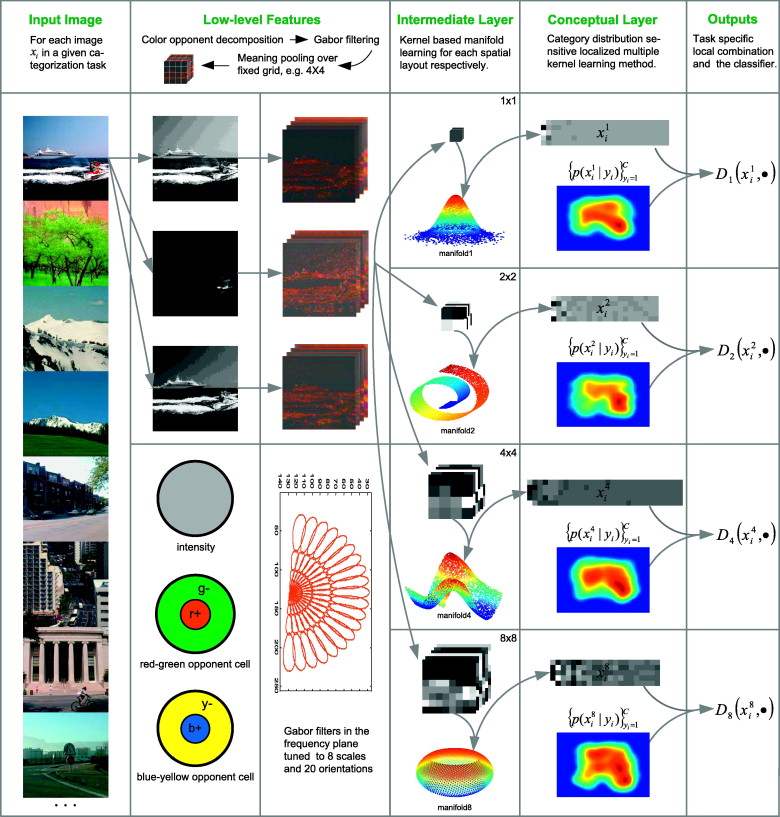
Fig.1 Framework overview: a color input image is first transformed to a vector through a series of low-level feature extraction strategy. At the next intermediate layer, the intrinsic manifold of feature vectors from current task is analyzed by kernel PCA method. CDS-LMKL is performed at the conceptual layer to yield the optimal task oriented resolution for spatial layout and the final classifier.
A scene image is provided that is a possible mechanism on how the human brain learns to encode the gist information includes both a bottom-up process for perceptual features and a top-down procedure for conceptual information.
For the first attribute of biological gist, mean pooling of biologically relevant Gabor features on fixed 4 × 4 grid proposed by Oliva and Torralba has researched promising results. It is by refining the low-level features in several biological plausible ways, extending the spatial layout to multiple resolution and followed by perceptually meaningful manifold analysis for a set of multi-resolution biologically inspired intrinsic manifold spatial layouts (BMSLs).
To the second attribute, it is embodied as learning the combination of BMSLs of multiple resolution with respect to their optimal discriminative invariance trade-off for the task at hand. And then it is cast in the SVM based localized multiple kernel learning (LMKL) framework, by which the kernel of each scene gist is approximated as a local combination of kernels associated to multi-resolution BMSLs.
By exploring the task specific category distribution pattern over BMSL, the local model as a category distribution sensitive (CDS) kernel is defined; this can accommodate both the diverse individuality of specific BMSL and the universality shared within the whole category space. Using CDS-LMKL, both the optimal resolution for spatial layouts and the final classifier can be efficiently obtained in a joint manner shown in Fig.2.
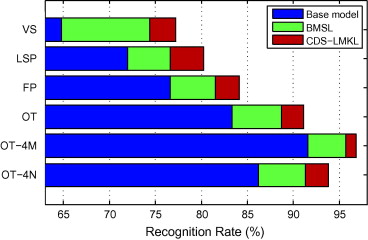
Fig. 2 The performance gains achieved by single optimal BMSL to the base model and by CDS-LMKL based combination of multiple BMSLs to single optimal BMSL.
As shown in Fig. 2, compared to single optimal BMSL, CDS-LMKL (p > 1) based multiple BMSLs can further improve the performances by around 3–4%.
BT-Gist on four natural scene databases and one cluttered indoor scene database with a range of comparison are evaluated: from different MKL methods, to various biologically inspired models and BoF based computer vision models. CDS-LMKL leads to better results compared to several existing MKL algorithms. Given the two biological attributes that the framework has to follow, BT-Gist, despite its holistic nature, outperforms existing biologically inspired models and BoF based computer vision models in natural scene classification, and competes with the object segmentation based ROI-Gist in cluttered indoor scene classification.
This research result was published online http://www.sciencedirect.com/science/article/pii/S107731421200135X and on the recently issued Computer Vision and Image Understanding (Vol. 117, No. 1, Pages 76–95, 2013).